Creating a Deep Learning Framework from Scratch in C++
Table of Contents
Prerequisites
You might be interested in my related articles on Matrix Multiplication and Concurrency before reading further!
Introduction
My goal for this project was to train a simple 2-layer Multi-layer perceptron by creating a Deep Learning Framework.
Typically representing models with a Dynamic Acyclic Graph (DAG) provides a better user debugging experience, so data and calculations had to flow at runtime.
So, I gave myself the following restrictions:
- Learn and incorporate as many design patterns and C++20 features as I could
- Use little to no dependencies in my code
This is what a sample training loop might look like with my library:
constexpr double LEARNING_RATE = 0.001;
constexpr double TRAINING_EPOCS = 300;
auto ma = NeuralNetwork::Computation::Graph::TensorConstructor::create(
Matrix::Rows(1),
Matrix::Columns(2000));
auto ground_truth = NeuralNetwork::Computation::Graph::TensorConstructor::create(
Matrix::Rows(1),
Matrix::Columns(10));
NeuralNetwork::Sequential model;
model.add(std::make_unique<NeuralNetwork::Layer>(
std::make_unique<NeuralNetwork::MatrixMultiplyStep>(Matrix::Rows(2000), Matrix::Columns(1000)),
std::make_unique<NeuralNetwork::AddStep>(Matrix::Columns(1000))
));
model.add(std::make_unique<NeuralNetwork::ActivationFunctions::ReLU>());
model.add(std::make_unique<NeuralNetwork::Layer>(
std::make_unique<NeuralNetwork::MatrixMultiplyStep>(Matrix::Rows(1000), Matrix::Columns(10)),
std::make_unique<NeuralNetwork::AddStep>(Matrix::Columns(10))
));
auto CE = NeuralNetwork::Computation::Graph::TensorOp(Matrix::Operations::Metric::CrossEntropy{});
for (int i = 0; i < TRAINING_EPOCS; i++) {
auto out = model.forward(ma);
auto loss = CE(ground_truth, out);
loss->backwards();
for (auto it = loss->parameters().begin(); it != loss->parameters().end(); ++it) {
*it += -LEARNING_RATE * it.gradient();
}
}
Results
I demonstrated my network improving via it’s loss function, but I wanted more! I saw that Matrix Multiplication represented the majority of the neural network computation’s runtime, so I found it was critical to optimize.
This project utilized parallel code via OpenCilk. It is a superset of C++ for parallel programming, hence the specialized compiler needed.
Here I profiled the elapsed time for a 25M parameter operation:
Operation | Data Size | Time (ms) |
---|---|---|
Parallel Matrix Multiply Benchmark | [5000, 5000] x [5000, 5000] |
6,831,126 |
Cross Entropy Metric Benchmark | [5000, 1] x [5000, 1] |
34 |
Outer Product Benchmark | [5000, 1] x [5000, 1] |
63,514 |
Softmax Benchmark | [5000, 1] x [5000, 1] |
5 |
Hadamard Product Benchmark | [5000, 1] x [5000, 1] |
21 |
Matrix Transpose Benchmark | [10000, 9000] |
659,166 improved to 66,771 |
In the end, I achieved a 142x and 10x speedup on MM and transpose ops by implementing Parallel Divide and Conquer algorithms and representing matrices in row-major order for reduced cache misses.
Design
I did this by encapsulating data in tensors, which were instantiated with a factory pattern and used in tandem with matrix operators, which were wrapped in a decorator object and together coupled with a global object to hold the graph’s edges.
- Each node contains a statically built finite state automata, used in tandem with traversal policies, such as gradient descent to update state.
- Additionally, iterator design patterns were used to decouple different traversal algorithms from the graph itself
Debugging
Just to add my own two cents to the build versus buy argument… planning ahead and choosing a mature library with linear algebra primitives would have been a smart choice for sure. It was pretty tough debugging large input matricies.
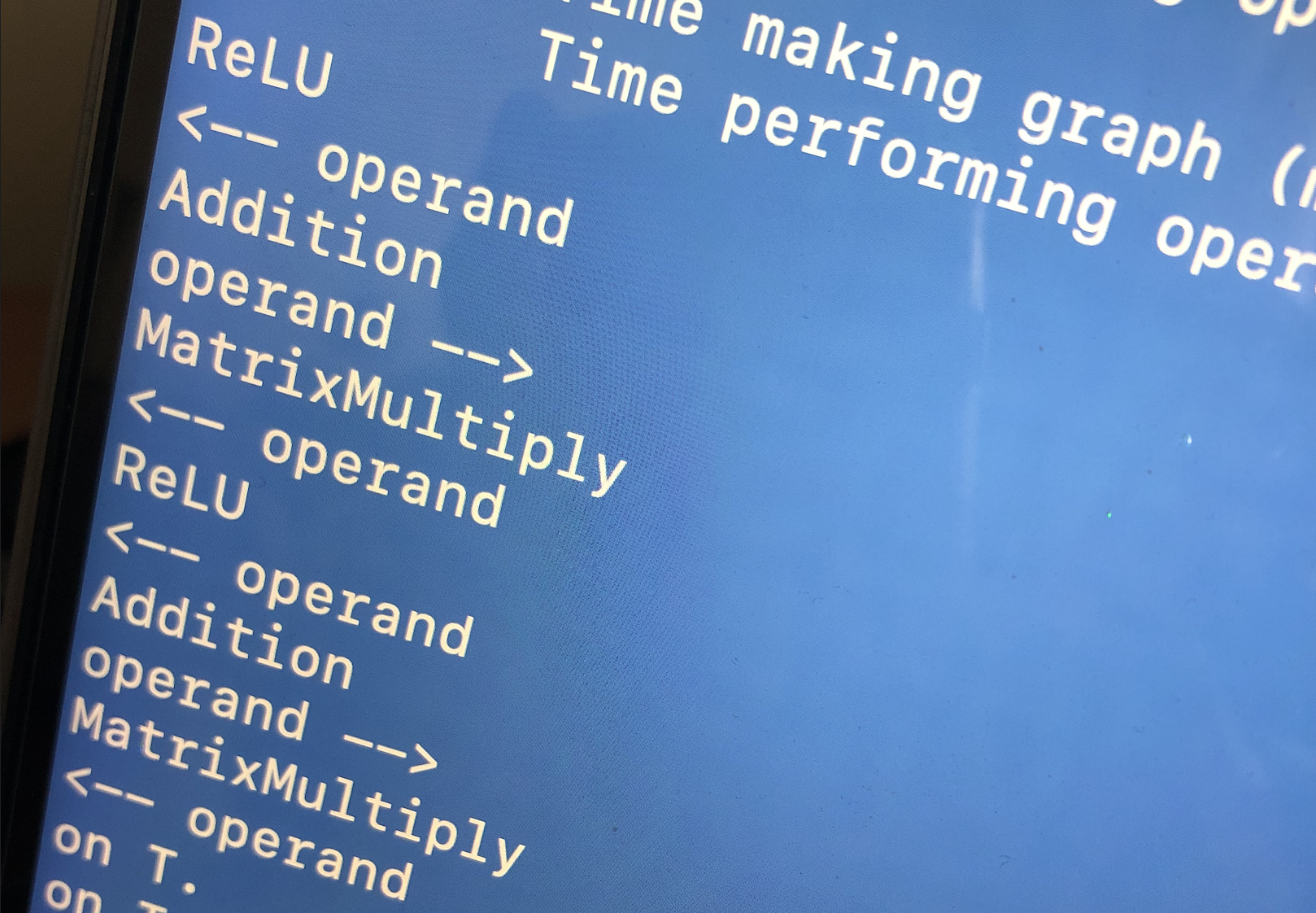
Figure 1: Terminal Output Demonstrated Order of Operations: To show me the way, print statements indicated that ReLU came before addition, which came before a Matrix Multiplication and so forth
Conclusion
This project came at a very personal time in my life. A lot of uncertainty was going on with my family, but I woke up every day programming to keep me going. I learned so much and used these learnings as I prepared for my next internship.
- Here’s a link to the repository if you’re interested: https://github.com/alejandroarmas/Wirikuta